Materials informatics – the use of data and AI methods to better understand the use, selection, development, and discovery of chemicals and materials – is one of the most visible and exciting ways in which digital transformation is impacting the chemicals and materials industry. The general hype surrounding AI has passed to MI, with startups raising millions over the past three years and leading players promising a revolution in the way that the industry operates. Despite the hype, the actual role of these technologies remains somewhat murky, especially for the more disruptive approaches. The major reasons behind this include the lack of data volume, format, and infrastructure, as well as cultural resistance. That said, many global companies have set their MI strategies by working with a startup, forming their own MI division, or joining a consortium. These recent activities indicate strong momentum, which will continue at an accelerated speed.
There is no consensus on the “right” way of defining MI applications. Based on our research and conversations with key stakeholders, we divide MI’s applications into two major types and believe this categorization is the simplest way of representing the MI space. The two categories are optimization of existing chemical and/or material structures and discovery of wholly new chemicals and materials, including the synthetic route. All optimization cases, regardless of the specific context, can be viewed as multivariable optimization problems where the goal is to find the best set of parameters corresponding to the features to be optimized, such as the type of chemicals/materials, processing, structure, properties, or performance. On the other hand, discovery cases often include the generation of new chemical structures with proposed synthetic routes to make them. To understand the landscape of major players in these two applications, we listed major MI players from corporates, small-to-medium enterprises (SMEs), and research institutes and broke down these players by geography. Through this analysis, we identified the general trend of MI to help companies find the right direction for building an MI strategy.
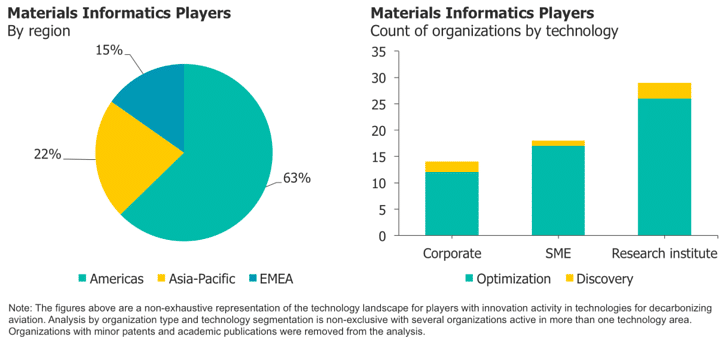
Our Data Indicates That the Americas Have the Highest Number of Mi Players, and Most of Them Are U.S.-based.
Leading companies include Schrödinger, Citrine Informatics, Kebotix, and Uncountable. Canada has the highest number of new MI startups, such as Phaseshift Technologies and AI Materia. Most of the Asia-Pacific players are global Japanese chemicals and materials companies. As we mentioned in a recent insight, Japanese companies are the most open to MI, and many of them have publicly announced their MI strategies. It’s certain that there are many more chemical companies with active MI efforts that have not been publicly disclosed, especially in the U.S. and EU.
Research Institutes’ Leading Role Indicates That Mi Is Still at an Early Stage.
AI is a fast-moving space, and many major AI algorithms have yet to be applied to chemicals and materials. Most research institutes focus on using newly developed algorithms in solving optimization-related use cases. The key players are Stanford University, Northwestern University, MIT, the National Institute of Standards and Technology (NIST), the National Institute for Materials Science (NIMS), the University of Cambridge, and the University of Toronto. Moreover, the comparison of corporations and SMEs indicates that more global corporations will engage with MI in the near future, especially in the Americas and EMEA.
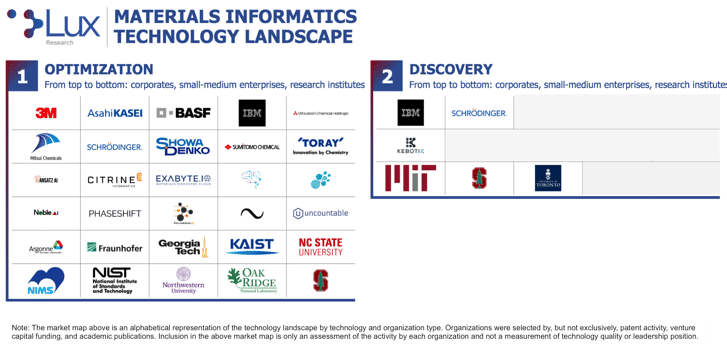
Most Mi Activities Are in Optimization with Traditional Data Science Methods.
This is due to the data challenges in the chemicals and materials industry. The industry not only lacks adequate volumes of data but also does not have one single format (or even a few formats) for data. What’s more, data often resides everywhere within a global company, and centralizing it is a major effort. With these factors and the expense of acquiring additional data, the chemicals and materials sector deals with “small and sparse data” the most. As a result, traditional statistical methods (rather than the much-hyped deep learning) often work better for MI. Often, the best data is available for optimization problems, leading most groups to work on such problems.
Most Players Belong to the Optimization Category, Targeting Two Specific Applications.
Optimization of polymer-based formulations has the highest commercial interest and is increasingly technically feasible. Recent computational methods have been used to replace experiments in this application, speeding up the process of data generation. The second application is multiphase alloy development. As we have a much longer history in metallurgy than in other materials, much more data is available for building phase diagrams. With AI tools, it becomes relatively practical to predict phase diagrams and/or phase behaviors of a given multicomponent alloy, enabling goal-driven alloy design.
Discovery Represents a New Wave of Mi, but Major Barriers Remain.
The most mature approaches today focus on small molecules and drug development. A typical example uses autoencoders to generate virtual molecule libraries for model training to select desired properties. After several rounds of screening by algorithms and human experts, it is possible to have a few testable chemical candidates for target properties. After the best candidate is selected, researchers can plan synthetic routes for production. While this method works on small molecules, it is still challenging to use on macromolecules like polymers, as there is not a satisfactory way to notate polymers on a large, deep learning-ready scale.
Many academic research groups have optimization technologies that are not yet commercialized. Top researchers include Dr. Taylor Sparks, Dr. Milad Abolhasani, Dr. Elizabeth A. Holm, and Dr. Klavs Jensen. In the next five years, more startups will emerge based on research institutes, and the key focus will be optimization, especially of polymers. As for discovery, many more research groups will begin to work on nonpolymeric chemicals and materials, likely using generative adversarial networks (GANs). Some of the work will look similar to what has been achieved in the small-molecule pharmaceutical space – with companies like AstraZeneca leading the efforts. Lastly, lab automation has emerged as a hardware solution for MI – creating the concept of the “autonomous lab.” This trend will continue in the near future but will not catch up with the pace of pure MI applications.